Introduction to AI in Engineering
Artificial Intelligence Applications in Engineering have emerged as a revolutionary force within the engineering domain, offering unparalleled opportunities for innovation and efficiency. At its core, AI encompasses a wide range of technologies, including machine learning, neural networks, and data analytics, each contributing uniquely to the engineering landscape.
Machine learning, one of the most prominent branches of AI, involves the development of algorithms that enable computers to learn from and make decisions based on data. This capability is particularly beneficial in engineering, where vast datasets are often generated. By leveraging machine learning, engineers can identify patterns, predict outcomes, and optimize processes, thus enhancing overall productivity and reducing costs.
Neural networks, inspired by the human brain’s structure, form the backbone of many AI systems. These networks consist of interconnected nodes that process information in a manner akin to human neurons. In engineering, neural networks are employed to solve complex problems, such as image and speech recognition, system modeling, and fault detection. Their ability to handle non-linear relationships and large volumes of data makes them invaluable tools in various engineering disciplines.
Data analytics, another critical component of AI, involves the systematic computational analysis of data. Engineers utilize data analytics to extract meaningful insights from raw data, facilitating informed decision-making and strategic planning. Through advanced analytics, engineers can monitor system performance, predict maintenance needs, and improve design processes, thereby driving innovation and efficiency.
The transformative potential of AI in engineering cannot be overstated. By integrating AI technologies, engineers can tackle challenges that were previously insurmountable, streamline operations, and foster a culture of continuous improvement. As we delve deeper into specific applications of AI in engineering, it becomes evident that AI is not just a tool but a catalyst for groundbreaking advancements in the field.
Click here to buy your best AI Materials using this affiliate link
Artificial Intelligence Applications in Engineering for Design and Simulation
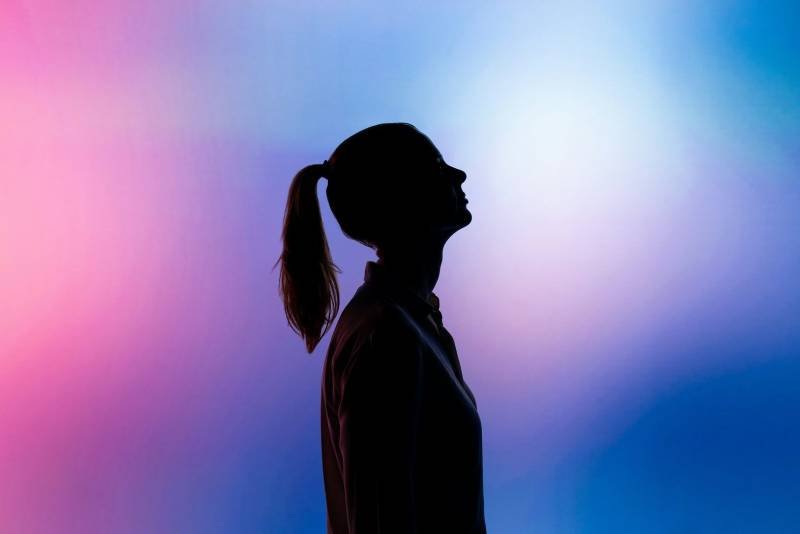
Artificial Intelligence (AI) has become a transformative force in the engineering domain, particularly in the stages of design and simulation. One of the most notable applications of AI in design is generative design. This innovative approach leverages AI algorithms to generate optimized design solutions based on a set of input parameters such as material properties, manufacturing methods, and performance criteria. Engineers can specify constraints and objectives, and the AI system then explores a vast design space to propose solutions that might not be intuitively obvious to a human designer. For instance, generative design has been employed in creating lightweight structures in aerospace engineering, leading to significant material savings and improved performance.
In the realm of simulation, AI enhances the predictive capabilities of traditional simulation tools. AI-driven simulation tools are adept at forecasting how a design will perform under different conditions, such as varying loads, temperatures, and environmental factors. These tools use machine learning models to analyze historical data and predict outcomes with high accuracy. For example, in automotive engineering, AI-powered simulations can predict the crashworthiness of a vehicle design, allowing engineers to make necessary adjustments early in the design process. This proactive approach not only accelerates the development cycle but also mitigates the risk of costly design flaws.
The integration of AI in design and simulation offers several compelling benefits. Firstly, it significantly increases efficiency by automating complex and time-consuming tasks, allowing engineers to focus on higher-level problem-solving. Secondly, AI-driven processes often lead to reduced costs by optimizing resource utilization and minimizing the need for physical prototypes. Lastly, AI fosters enhanced innovation by enabling the exploration of unconventional design solutions that human designers might overlook. By leveraging AI, engineering projects can achieve superior performance, sustainability, and overall quality, thereby driving the industry forward.
Click here to buy your own AI Materials using this affiliate link
Predictive Maintenance and Artificial Intelligence Applications in Engineering
Predictive maintenance, driven by artificial intelligence (AI), is revolutionizing how industries manage their equipment and machinery. By leveraging advanced AI algorithms, companies can anticipate equipment failures before they occur, thus optimizing operational efficiencies and extending the life of critical assets. This predictive capability is particularly transformative in sectors such as manufacturing, aerospace, and automotive, where machinery downtime can result in significant financial losses and operational disruptions.
AI’s role in predictive maintenance primarily involves analyzing vast amounts of data collected from various sensors embedded within the machinery. These sensors continuously monitor parameters like temperature, vibration, and pressure, generating real-time data streams that are fed into AI systems. Through machine learning models and data analytics, AI can identify patterns and anomalies that may indicate potential failures. This proactive approach allows maintenance teams to address issues before they escalate into major problems, thus minimizing unscheduled downtime and maintenance costs.
In the manufacturing sector, for instance, AI-driven predictive maintenance is employed to monitor assembly lines and machinery. Companies like Siemens have integrated AI to predict failures in their manufacturing processes, resulting in a significant reduction in unexpected downtimes and maintenance expenses. Similarly, in the aerospace industry, AI is used to forecast the maintenance needs of aircraft engines. GE Aviation utilizes AI analytics to predict component failures, ensuring timely maintenance and reducing the risk of in-flight malfunctions.
The automotive industry also benefits from AI in predictive maintenance. Car manufacturers such as BMW use AI to monitor the health of their production equipment. By predicting the failure of critical components, they can schedule maintenance during non-peak hours, thereby maintaining continuous production flow and enhancing overall efficiency.
These industry case studies underscore the profound impact AI can have on predictive maintenance. By harnessing the power of AI to analyze sensor data and forecast maintenance needs, industries can achieve higher reliability, reduced costs, and extended machinery lifespans. As AI technology continues to evolve, its application in predictive maintenance is expected to become even more sophisticated, offering unprecedented levels of operational efficiency and asset management.
AI in Quality Control and Inspection
Artificial Intelligence (AI) is revolutionizing the field of quality control and inspection within engineering, bringing unprecedented levels of accuracy and efficiency. One of the cornerstone technologies driving this transformation is computer vision. Computer vision systems, powered by AI, can analyze visual data from cameras and other sensors to detect defects and anomalies in products. This capability is crucial in manufacturing environments where the consistency and quality of products are paramount.
Machine learning algorithms further enhance these systems by enabling them to learn from historical data and improve their defect detection capabilities over time. For instance, AI models can be trained on vast datasets of images showing defective and non-defective products. Over time, these models become adept at recognizing subtle defects that might be missed by human inspectors or traditional inspection methods. This learning process not only heightens the accuracy of inspections but also reduces the time required to identify issues.
The advantages of AI-driven quality control extend beyond mere accuracy. Speed is a significant benefit, as AI systems can process and analyze data much faster than human inspectors. This rapid analysis means that defects can be identified and addressed in real-time, minimizing the risk of faulty products reaching the market. In industries where time is critical, such as automotive and electronics manufacturing, this speed can translate to substantial cost savings and enhanced product reliability.
Moreover, AI systems can handle large volumes of data, making them scalable solutions for quality control in mass production environments. As these systems continue to evolve, they are becoming increasingly adept at handling complex inspection tasks, such as identifying micro-defects or assessing the structural integrity of materials. This scalability ensures consistent quality across all units produced, thereby enhancing overall product standards.
In summary, the integration of AI in quality control and inspection offers significant improvements in both accuracy and efficiency. By leveraging computer vision and machine learning algorithms, AI systems not only detect defects with high precision but also operate at speeds unattainable by traditional methods. As these technologies continue to advance, the future of quality control in engineering looks increasingly promising.
Artificial Intelligence Applications in Engineering for Supply Chain Management
Artificial Intelligence (AI) is revolutionizing supply chain management within the engineering sector by enhancing demand forecasting, inventory management, and logistics optimization. By leveraging AI, companies can anticipate supply chain disruptions and respond proactively, thereby improving overall efficiency and reliability.
One of the most significant applications of AI in supply chain management is demand forecasting. Traditional forecasting methods often rely on historical data and can be limited in their accuracy. AI, on the other hand, utilizes advanced algorithms and machine learning techniques to analyze large datasets, identify patterns, and predict future demand with a higher degree of precision. For example, AI systems can incorporate real-time data from social media trends, weather forecasts, and economic indicators, allowing companies to adjust their production schedules and inventory levels more effectively.
Inventory management is another area where AI is making a substantial impact. AI-powered systems can monitor inventory levels in real-time, predict stock shortages, and recommend optimal reorder points. This not only reduces the risk of stockouts and overstock situations but also minimizes holding costs. For instance, AI can analyze sales data, lead times, and supplier performance to ensure that inventory is maintained at optimal levels, thereby reducing waste and improving cash flow.
Logistics optimization is also being transformed by AI. By analyzing data from various sources such as GPS, traffic patterns, and delivery schedules, AI can optimize routes, reduce fuel consumption, and improve delivery times. This is particularly valuable in engineering projects where timely delivery of materials and components is crucial. Companies can use AI to predict potential disruptions such as traffic jams or adverse weather conditions and adjust their logistics plans accordingly. For instance, a construction company might use AI to reroute deliveries in real-time to avoid delays and ensure that projects stay on schedule.
Moreover, AI helps companies to anticipate supply chain disruptions by providing early warnings and actionable insights. Advanced AI systems can detect anomalies and predict potential issues such as supplier failures or geopolitical risks, enabling companies to take proactive measures. This predictive capability enhances the resilience of the supply chain, ensuring that engineering projects are completed on time and within budget.
The integration of artificial intelligence (AI) with robotics in engineering has revolutionized various industrial sectors, offering unprecedented levels of efficiency and precision. AI-powered robots are now playing crucial roles in manufacturing, construction, and other engineering fields, performing tasks that range from assembly and welding to inspection and maintenance.
Artificial Intelligence Applications in Engineering for Manufacturing
In the manufacturing sector, AI-driven robots are indispensable for assembly line tasks. These robots are equipped with advanced sensors and machine learning algorithms that enable them to perform intricate tasks with high accuracy. For instance, in automotive manufacturing, AI robots handle the assembly of small components, ensuring that each part fits perfectly, thereby reducing the margin for error and enhancing the overall product quality.
Artificial Intelligence Applications in Engineering for Construction
Construction is another field where AI-powered robotics are making significant strides. Robots equipped with AI can perform complex tasks such as bricklaying, welding, and even 3D printing of building components. These robots can operate in hazardous environments, reducing the risk to human workers and improving safety standards on construction sites. Moreover, AI algorithms can optimize the robots’ operations, ensuring that tasks are completed faster and more efficiently.
Inspection and Maintenance
AI-driven robots are also widely used for inspection and maintenance tasks in various engineering domains. In the oil and gas industry, for example, robots with AI capabilities can inspect pipelines and detect potential issues such as leaks or corrosion. These robots can analyze vast amounts of data in real-time, providing engineers with actionable insights and enabling predictive maintenance. This not only reduces downtime but also prevents catastrophic failures and enhances operational safety.
Overall, the integration of AI with robotics in engineering offers numerous benefits. Improved precision is one of the most significant advantages, as AI algorithms enable robots to perform tasks with minimal errors. Additionally, productivity is enhanced as robots can work continuously without fatigue, completing tasks faster than human workers. Lastly, the use of AI-driven robots improves workplace safety by taking over dangerous tasks, thereby reducing the risk of accidents and injuries.
Challenges and Ethical Considerations
The integration of Artificial Intelligence (AI) in engineering brings forth a multitude of challenges and ethical considerations that must be meticulously addressed. One of the primary concerns is data privacy. AI systems often require vast amounts of data to function effectively, raising significant questions about how this data is collected, stored, and utilized. The potential for misuse or unauthorized access to sensitive information necessitates robust data protection measures and stringent privacy policies to safeguard individual and organizational data.
Another critical issue is job displacement. As AI technologies continue to evolve, there is a growing concern that they may replace human labor in various engineering roles. While AI can enhance efficiency and productivity, it also poses a threat to job security for many professionals within the industry. Addressing this challenge requires a balanced approach that includes re-skilling and up-skilling initiatives to prepare the workforce for new, AI-centric roles, thereby mitigating the adverse effects on employment.
Transparency in AI decision-making processes is another significant ethical consideration. AI systems, especially those utilized in engineering, must be transparent in their operations to foster trust and accountability. This involves making the decision-making algorithms and data sources understandable and accessible to stakeholders. Transparent AI ensures that decisions made by these systems are explainable and can be scrutinized, which is crucial for maintaining ethical standards and public trust.
Furthermore, the development of ethical guidelines and regulatory frameworks is imperative to ensure responsible AI deployment in engineering. These frameworks should encompass principles such as fairness, accountability, and non-discrimination, guiding the development and implementation of AI technologies. Regulatory bodies must work collaboratively with engineers, AI developers, and other stakeholders to create comprehensive policies that address the ethical implications of AI, promoting its use in a manner that benefits society while minimizing potential harms.
In conclusion, addressing the challenges and ethical considerations associated with AI in engineering is essential for its sustainable and responsible integration. By prioritizing data privacy, mitigating job displacement, ensuring transparency, and developing robust ethical guidelines, the engineering field can harness the transformative potential of AI while upholding the highest ethical standards.
Future Trends and Conclusion
As we look ahead, the role of artificial intelligence (AI) in engineering is poised to expand even further, driven by a host of emerging technologies and potential advancements. One significant trend is the integration of AI with the Internet of Things (IoT), enabling smart sensors and devices to communicate and collaborate in real-time. This fusion promises to enhance predictive maintenance, optimize resource management, and improve overall system efficiency in various engineering sectors.
Another promising avenue is the use of AI-driven generative design. This technology leverages machine learning algorithms to create optimized designs based on defined parameters and constraints, potentially revolutionizing the field of product development. Engineers can now explore a multitude of design alternatives rapidly, leading to innovative solutions that were previously unimaginable.
Furthermore, advancements in natural language processing (NLP) are set to make AI systems more intuitive and user-friendly. This will allow engineers to interact with complex systems using simple, conversational language, thereby reducing the learning curve and making sophisticated tools more accessible to a broader audience. NLP can also facilitate better data interpretation and decision-making through enhanced analysis of technical documents and reports.
Quantum computing is another frontier that holds immense potential for AI in engineering. With its ability to process vast amounts of data at unprecedented speeds, quantum computing could enable more accurate simulations, complex problem-solving, and the development of new materials and technologies. Although still in its nascent stages, ongoing research and development in this area could lead to groundbreaking applications in the near future.
In conclusion, the transformative impact of AI on engineering is undeniable. From improving efficiency and accuracy to fostering innovation and creativity, AI applications are reshaping the landscape of engineering. Staying informed about these ongoing developments is crucial for professionals in the field, as the rapid pace of technological advancement promises to bring about even more revolutionary changes. By embracing these future trends, engineers can continue to push the boundaries of what is possible, driving progress and creating a smarter, more efficient world.
Buy Your Best AI Applications material
[…] for Professional Use Essential Tech Tips to Avoid Lightning Strikes During a Thunderstorm Artificial Intelligence Applications in Engineering Best Tech for 2024 Productivity Hacks and Tips Best Tech Ultimate Guide iPhone vs Samsung Best Tech Features, […]